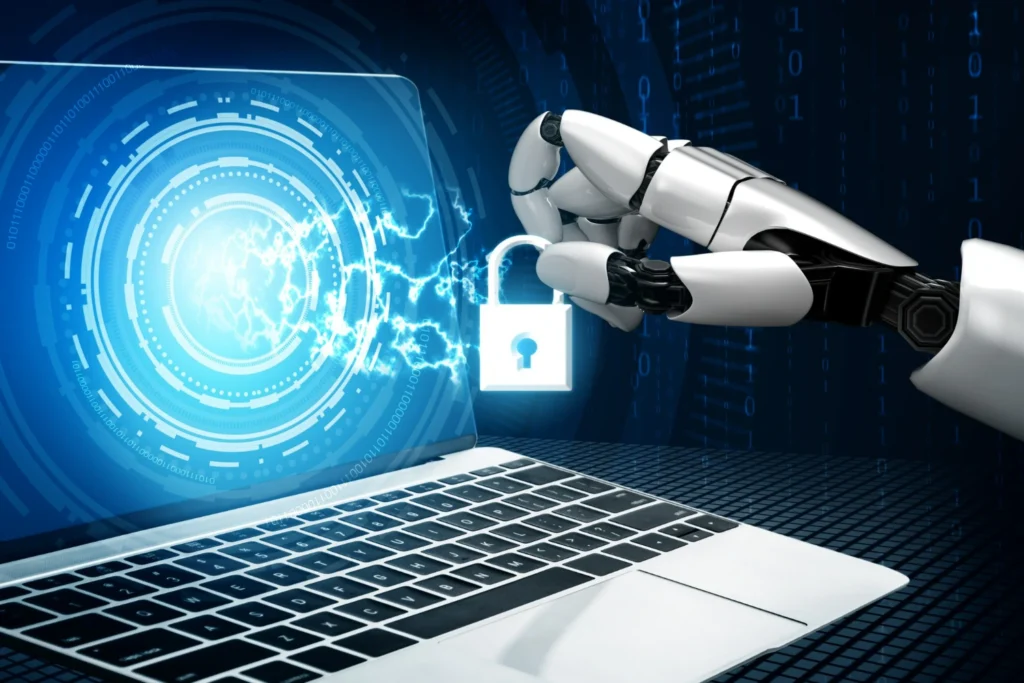
Agentic AI – A Boon or Threat for Enterprise Cybersecurity?
An interesting session with the Chief Information Security Officer (CISO) of one of our global customers on application of new age technologies to strengthen their Cybersecurity implementations has suddenly took a turn when he has passed a query to me “ is Agentic AI pose a threat for my Cybersecurity initiatives? ”. Noting down my POV on this interesting ,curious and at the same very relevant scenario for global enterprises.
Agentic AI, the latest entrant into global AI landscape can seamlessly interact with enterprise systems for inputs which can enable real time event correlation and subsequent decision making on the best possible course of resolution . With its autonomy and outcome-oriented approach, Agentic AI has tremendous potential to disrupt positively the enterprise Cybersecurity landscape. Its ability to learn, adapt, and act independently makes it a powerful tool for detecting and responding to threats depending on how its implementation and Governance is done.
While it has the potential to significantly enhance threat detection and response capabilities due to its autonomous decision-making, it also carries risks like potential false positives, misinterpretations, and vulnerabilities if not properly designed and monitored, requiring a careful balance between automation and human oversight.
Agentic AI is designed to act as an autonomous agent capable of assessing its environment, making decisions, and acting upon so as to achieve specific goals. In Cybersecurity, AI Agents can analyze large datasets, identify patterns, and generate actionable insights. Traditional automation techniques are based on predefined rules that govern the behavior of a system, while AI agents run on training data and instructions.
Some of the highlights of Agentic AI based Cybersecurity implementations are :
Rapid threat detection aiding UTM
Unified threat management (UTM) is a network security system that combines multiple security features into one device or service. It helps protect networks from a variety of threats, including malware, viruses, and network attacks. Agentic AI analyze vast amounts of data in real-time, identifying suspicious patterns and potential threats much faster than human analysts, allowing for quicker response times.
Automated Remedial Actions
Agentic AI can automatically take mitigation actions like isolating compromised systems or blocking malicious traffic, reducing the time needed for human intervention. AI agents can monitor networks and systems around the clock, providing continuous protection without the need for human oversight.
Adaptive Threat Analysis:
Agentic AI can learn and adapt to evolving threat landscapes, constantly refining its detection algorithms to identify new attack vectors. It can also n analyze historical data and trends to predict potential vulnerabilities or attack vectors thereby bolstering defenses before an attack occurs.
Threat Intelligence
Agentic AI can aggregate and analyze data from various sources to anticipate new threats and provide intelligence on emerging risks. They can use machine learning models to understand the normal behaviour of users and devices, and flag deviations as potential threats like insider threats, compromised user accounts etc
Incident Investigation and Forensics
Agentic AI using advanced techniques like deep learning, can reduce false alarms by more accurately distinguishing between normal activities and actual threats. It can quickly sift through large volumes of data during an investigation, identifying key evidence and helping security teams understand the scope and impact of an attack.
Adaptive Défense Mechanisms
Agentic AI can adapt to new attack strategies and tactics by learning from emerging threat data, ensuring that defenses are always evolving in response to new risks. In cases of a detected attack, AI can automatically take defensive actions reducing human efforts.
While Agentic AI provide powerful tools for improving Cybersecurity, organizations must be aware of the associated risks. These include vulnerabilities to adversarial attacks, issues of bias, lack of transparency, the potential for over-reliance, privacy concerns, and the challenge of adapting AI to new and novel threats. Few of the real-life scenarios where Agentic AI can cause potential threats for enterprise Cybersecurity implementations are
False Alerts
Due to the complexity of data analysis, Agentic AI might generate false alerts or miss real threats, leading to unnecessary disruptions or missed opportunities to address critical issues
Malicious attacks
Malicious actors could potentially exploit Agentic AI for sophisticated attacks by manipulating its decision-making process. In certain cases, attackers might inject malicious data into the training process, altering the AI model’s behavior, making it more susceptible to exploitation
Lack of transparency
The decision-making process of complex Agentic AI systems can be difficult to understand, making it challenging to debug errors or explain why certain actions were taken.
Balancing the use of AI with human oversight, continuous monitoring, and regular updates to the system is essential for managing these risks effectively.
Data Privacy Concerns
Agentic AI in cybersecurity often require access to sensitive data to identify threats. Improper handling of this data or a data breach involving an AI-driven security system could lead to significant privacy violations.
Design considerations for Agentic AI in Cybersecurity
Agentic AI has the potential to be a significant asset for enterprise Cybersecurity, but it is crucial to implement it responsibly with adequate safeguards and human oversight to mitigate potential risks and maximize its benefits .Below are some critical design considerations while employing Agentic AI in enterprise class cyber implementations
Human-in-the-loop (HITL) approach
Always ensure that human operators can review and validate critical security decisions made by Agentic AI systems. AI agents should be designed to work alongside human security experts, not replace them. The system should alert, support, and provide recommendations to human analysts, allowing them to make final decisions, especially in complex or ambiguous situations.
User Feedback loop
Design the system to learn from human feedback on the decisions it makes. This creates a continuous feedback loop where both AI and human expertise contribute to improving the system’s accuracy and effectiveness.
Data Privacy
For handling sensitive data, such as personally identifiable information (PII) or proprietary business data, need to ensure that the system follows data privacy regulations and employs encryption, anonymization, or other privacy-preserving techniques when processing data.
Scalability and Performance
Agentic AI must be scalable to handle large volumes of data, especially for organizations with massive networks and systems. This involves choosing suitable AI models and architectures that can scale with the increasing complexity and volume of cybersecurity data. Also, it should be capable of processing data and responding to threats in near real time
Regular monitoring and evaluation
Continuously monitor the performance of Agentic AI systems and make adjustments as needed to maintain effectiveness.
Designing Agentic AI for Cybersecurity requires a multifaceted approach to ensure that they are effective, secure, and adaptable in the face of evolving cyber threats. These considerations highlight the importance of data quality, system performance, transparency, adaptability, and integration with human and organizational processes. By addressing these factors, organizations can build AI-driven Cybersecurity systems that are not only powerful but also resilient and trustworthy.
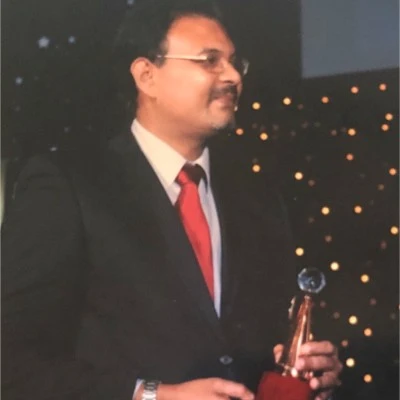
Sajeev Nair
CTO ( Tech & Digital) @ Digitide Solutions Ltd | “AI-First Digital Native Value Creator”
Currently Chief Technology Officer (Tech &Digital ) at Digitide Ltd, I have over 29 years of industry expertise in leading enterprise-class digital transformation initiatives in the areas of distributed ADM , Legacy Modernization, Digital Integration and Process automation globally across BFSI, CPG, Retail, Utilities, Travel ,Transportation & Airlines, Telecom and Healthcare domains. In my current role, responsible for the technology strategy, vision, P&L of Niche practices, sales enablement and innovations for the digital transformation services of Conneqt Digital. My core competencies include CX transformation solutions using leading platforms Adobe, Liferay, Optimizely, LCAP platforms Pega, Outsystems, Appian, Mendix, Microsoft PowerApps, hybrid integration platforms Mulesoft, Boomi, Tibco and 360-degree data platforms like Snowflake, Databricks, Azure Data Fabric, Cloudera, BI and visualization platforms like ThoughtSpot, Tableau, PowerBI, etc. GenAI / Agentic AI based domain use case catalogue creation and its effective implementation for our global customer landscape is my latest professional muse since last few quarters. We leverage these technologies to provide end-to-end enterprise transformation solutions for our customers in the US, ME, and India. Our mission is to enable our customers to achieve their business goals and outcomes through digital innovation and excellence.